Best Practices with Data in Spark
To help your team maximize its efforts, here are some best practices we recommend to manage and utilize your data from Spark.
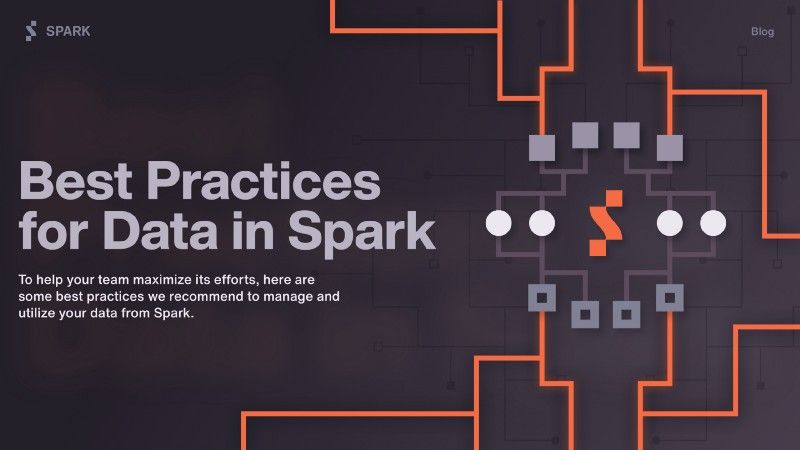
You’ve successfully launched your first project in Spark. Congratulations! Soon you’ll have a data set filled with customer information, demographic details and important insights that can be used to your advantage.
This data has the ability to enable you to make decisions to shape your future marketing and sales strategies, as well as bring you one step closer to a successfully sold out project.
We know that this level of data can be daunting and finding the best way to shape, organize and use it effectively can be a challenge. To help your team maximize its efforts, here are some best practices we recommend to manage and utilize your data from Spark.
Keep Your Data Relevant
The best practice we recommend for Spark users is to keep your data relevant. When it comes to data collection and analysis, it might feel like common sense to collect as much information for as many contacts as possible with the mindset that more data = more insights.
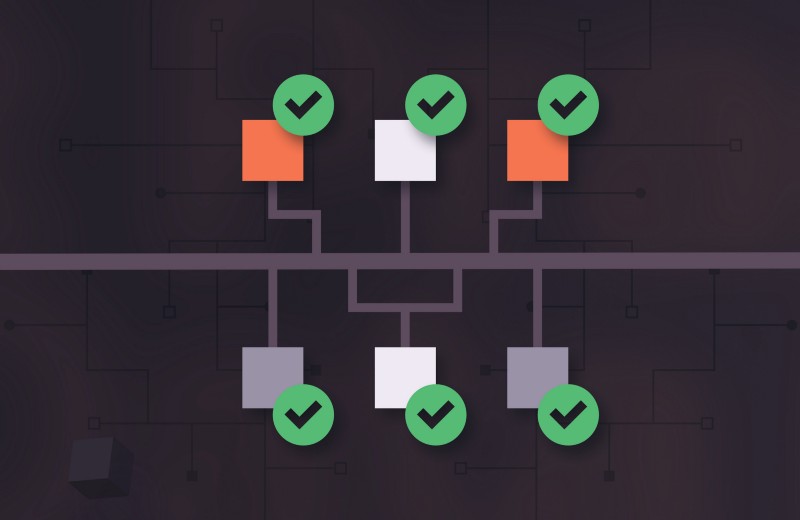
While sometimes true, your data risks being jumbled and full of unnecessary, overly-granular points. This could lead to the truly valuable insights becoming lost in the noise. Before beginning any data collection, we recommend first identifying what your goals are with your marketing, what is needed to achieve them and what data must be collected to contribute to those goals. Your marketing Key Performance Indicators should be your priority here, while contextual data points are only contributors to achieving these KPIs.
Another key aspect of keeping your data relevant is to keep your data up to date. Over time, the relevance of your data loses its integrity given that demographics, behaviours and socioeconomic factors continue to change. You wouldn’t want to rely on data from 5 years ago to make decisions today. Continuing to enter new contracts into Spark and keeping interactions up to date will allow you to populate and update your centralized data set and ensure you’re making more accurate strategic decisions.
Keep Your Data Clean
Another key best practice we recommend is to keep your data clean. This is twofold: 1) data standardization and 2) data integrity.
Data standardization is one of the most vital processes we recommend to all our clients. Creating rules and set terminology for data input will save significant headaches when you delve into reporting and data analysis. This applies doubly to customer-facing lead generation forms, with the risk of hundreds to thousands of registrants filling out forms with a multitude of inputs that mean the same thing.
Without a standardized format, your data will not be grouped correctly and lead to incomplete analysis. “BC” versus “British Columbia” may mean the same thing to you, but your data analysis program won’t feel the same. Save yourself the hassle and create standardized data input rules for your team and registrants at the outset. We highly recommend that our clients first determine the information they need and how they will be using it, then consult their Customer Success Manager to ensure they’re collecting it in the most efficient and effective way possible.
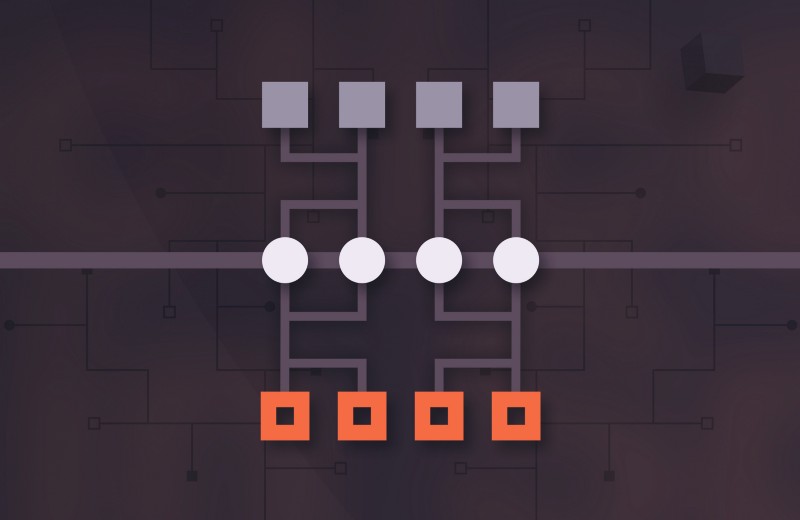
The second important tenant of keeping your data clean is data integrity. In the heat of a sales cycle, sales reps may make an error like accidentally forgetting to fill in certain forms or choosing the wrong drop down option. Building your upcoming marketing strategy upon this analysis could lead to inaccurate decision making and waste vital time, resources and money that could impede your future decisions and marketing efforts.
Programming fail-safe measures such as only accepting inputs from a list of pre-specified options or mandatory field rules will help eliminate the risk of errors such as typos and blank fields. For example, if a sales representative accidentally misses a field when filling out a customer profile, your pre-programmed fail-safe measures won’t allow them to save without filling out that missing field. Some errors may still slip through the cracks. As a result, periodic data sanitization is necessary to ensure that the structure that you’ve set in place is working and to fix any remaining errors in your data.
Watch out for Patterns
As you host more projects in Spark, you have the unique opportunity to grow and nurture your own distinctive competitive advantage. Many people forget that the purpose of collecting data in the first place is to generate insights. Finding ways to consistently make use of your data will help you find the best ways to collect and standardize it. Organized in a central location, your team will be able to pull relevant information from both the individual project level and company-wide level. Whether you want to compare demographics between two of your low-rise projects or see average income levels across all projects you’ve launched, Spark can allow your team to do so.
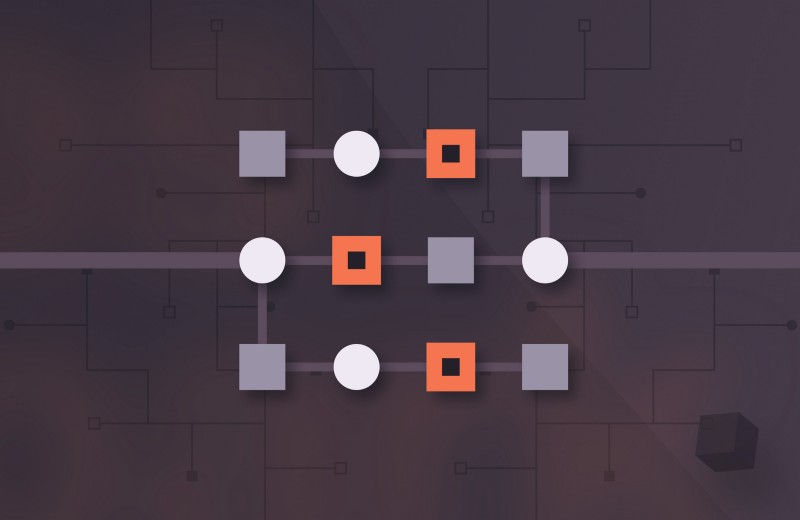
Together, you’ll be able to identify patterns and trends on both a macro and micro level, which can create a foundation for the success of future projects. Macro patterns, the most common trends to be found, typically relate to a large group’s demographic and psychographic characteristics. This includes patterns such as a significant number of leads coming from a specific neighborhood or buyers of a certain unit type sharing a similar income level. However, micro patterns should not be ignored; being able to identify trends amongst individuals may help predict single sales and identify possible opportunities for account based marketing.
Whether you find a bulk of individuals from a specific location purchasing your properties or identify a loyal buyer that has purchased from your team multiple times, the insights you can pull from your data can lead to dividends for your bottom line.
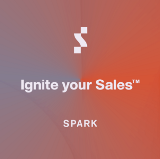